More Innovation:
Using Computing Power to Track and Project COVID-19’s Spread
Published: July 6, 2020
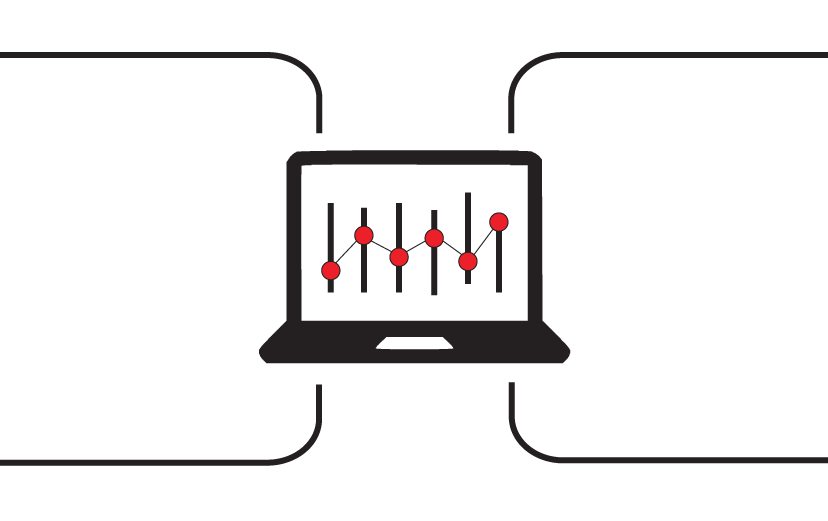
SUMMARY
- It is essential to build mathematical models that track and project COVID-19’s spread to help all levels of government make social policy decisions despite the difficulties and expenses.
- Generally, the more data sources these models include — such as population mobility and testing results — the more insightful they become.
- One modelling method that researchers commonly use is called Trajectory Matching which predicts the outcome of various mitigation strategies. However, it requires enormous amounts of computing resources.
- Ontario company, Distributive, is using their “Distributed Computer” to crowdsource computing resources from companies, universities, and even individuals for affordable Trajectory Matching.
- This enables communities at all levels to deploy these epidemiology models at a scale previously only available to the federal government.
- Distributive has been awarded a substantial grant from the federally backed Digital Technology Supercluster using their platform named “Project Looking Glass”.
- Project Looking Glass will be deployed across Canada in the coming weeks and months.
MAPPING (AND PREDICTING) THE SPREAD OF COVID-19
If you want to find the latest numbers on COVID-19, there’s no lack of resources available to the public.
Websites such as Worldometer are providing updated numbers on those who are infected in various countries. If you’re looking for something more geographical and detailed, Johns Hopkins University has created an online dashboard that allows you to see the live spread of COVID-19 on a world map. Even Google has taken part.
As much as these sources of information are interesting, they are not very helpful in predicting the further spread of COVID-19. To use these alone would be like driving while only looking in the rear-view mirror.
To combat COVID-19’s transmission, municipal, provincial, and federal governments need more than just the current numbers to make decisions. They need mathematical models that could predict where people are going to be infected and how likely they are to spread COVID-19 onto others.
Adding to the pressure, many COVID-free municipalities are quite reasonably urging re-opening, even as transmission continues elsewhere. Policymakers in these areas need to be able to prove it is safe to open businesses. For them, localized models that predict transmission in the community are key – the same kind of predictive models that only exist at the national and provincial level right now.
USING A ONE SIZE FITS ALL APPROACH
Currently, the government of Canada has had to use a “one size fits all” approach to combat COVID-19, closing all schools, businesses, recreational parks, and more. Though this strategy is effective for containing the spread, it can have more negative impacts than positive ones depending on the situation of various towns and cities.
Intuitively, it makes sense to close everything in large urbanized areas such as Toronto or Vancouver to stop the spread, but what about more rural areas such as Pickle Lake in Northern Ontario?
With a population under 500, this remote town may be at very low risk for a viral outbreak, yet thanks to provincial one-size-fits-all policies, all businesses are shut down possibly causing the small-town more damage than it would suffer from COVID-19. Beyond closed businesses, a general shutdown can also delay the delivery of vital medical, food, and other supplies which can lead to negative health and livelihood outcomes.

So, what would happen if Pickle Lake were to open back up? Would things go back to normal or would COVID-19 spread through this tiny community?
Unfortunately, without the right data and analysis techniques to answer these questions, using a “one size fits all approach” really is the best option. After all, the government has only a big picture view of how this situation looks – too big for treating each community case-by-case in such small detail.
But what if we had the right tools? What if there was a way to have full predictive abilities at the community-level, rather than just provincially and nationally? Maybe communities like Pickle Lake could open up and suffer neither ill effects from a shut-down nor COVID-19 itself.
Luckily, it’s possible to dive into that amount of detail.
USING TRAJECTORY MATCHING FOR MORE ACCURATE DATA
Using a method called Trajectory Matching, researchers and epidemiologists are looking to provide an accurate model of COVID-19’s transmission patterns.
Fundamentally, Trajectory Matching is a mathematical process that uses current and past data to build a model forecasting future growth and/or decline of disease. This model can then be adjusted to account for changes in the system, whether observed or predicted.
For example, if all previous data points had an exponential trend upward but something physically changed in the system (e.g. social distancing, which reduces the rate of spread or R0 of COVID-19), the Trajectory Matching model will take these changes into account to produce a new forecast of the future.
Of course, travel is an important factor. To account for this, anonymous transportation data from telecommunication corporations can be used to map the movements of people, which combined with infection and transmission patterns of COVID-19, can be used to visualize the predictions made using the epidemiology models.
With this data, researchers can predict the outcomes of almost any public health decision, seeing how it will affect public health and economic factors. These predictions can also go into specific detail, analyzing only certain provinces, counties, and even municipalities in Canada.

This information is not just used to predict the future spread of the virus. It can also be used to determine how vaccines should be rolled out, what businesses and areas should be opened first, and even when the next wave of the virus will occur.
Distributive, an award-winning start-up in Ontario, is leading the effort to build these models.
DISTRIBUTIVE & PROJECT LOOKING GLASS
Located in Kingston, Ontario, Distributive is assisting in mapping these transmission patterns using their own “plug and play” platform called Project Looking Glass.
Project Looking Glass is a collaboration between Distributive, Queen’s University, Saskatchewan Polytechnic, CENGN, and many more universities, companies, and cities. The goal is to create a powerful web-based platform that different jurisdictions can utilize to forecast the result of different decisions on the spread of COVID-19.
The models available through Looking Glass will make use of vast amounts of both open-source and proprietary data. For greater accuracy and relevance, municipalities can further integrate their datasets with those provided by the core platform. These could be anything from suspected cases to the number of healthcare workers that commute.
Looking Glass uses data and computation to enable confident decision-making. It does this by allowing researchers and epidemiologists to create Trajectory Matching models that predict the outcome of various decisions. Pickle Lake for example could simulate the expected transmission of COVID-19 in the community if it re-opened all stores and restaurants to normal capacity.



The downside of Trajectory Matching is that it requires large amounts of computational power due to the intense mathematical processes. Without enough computational resources, calculating just one version of a model can take days, weeks, or even months.
Although Looking Glass is innovative in how it integrates different datasets into a web application, its true advantage is how it puts inexpensive computing resources within reach. Without this, Trajectory Matching models would still take too long for timely decisions to be made.
This is where the Distributed Computer comes in.
WHAT IS THE DISTRIBUTED COMPUTER?
Created by Distributive, the Distributed Computer is an advanced and secure computing platform that allows owners to “rent out” and buyers to “purchase” underused CPU and GPU resources. The cash can later be withdrawn to their bank or used to purchase CPU and GPU resources for their projects.
The idea of creating a “marketplace” for computing – from supercomputers to smart TVs – is how Distributive can provide the smallest communities with first-class computing infrastructure. The Distributed Computer is how Pickle Lake can make informed decisions with the level of detail that used to only be available at the national and provincial levels.

Originally designed before the COVID-19 pandemic, the Distributed Computer is an improvement over current computing architectures. The same code can run on any device from supercomputers to smartphones, seamlessly integrating complex webs of software as no other technology can.

Competing products also require a combination of serious configuration and programming knowledge, dedicated infrastructure, and – most often – a large budget. The Distributed Computer removes this complexity on the user’s end with minimal setup and significantly reduces the cost of compute.
EYES ON COVID-19 RECOVERY
In May 2020, Distributive and its partners were awarded a $2.2 million grant from the government-sponsored Digital Technology Supercluster to build Project Looking Glass. The initiative is led by Distributive in partnership with Riskthinking.AI, Limestone Analytics, Queen’s University, aiSight, and Distributed Compute Labs. Project contributors include CENGN, Server Cloud Canada, Dymond, AMPD, PHEMI Systems, Krate Distributed Information Systems, and multiple municipalities in Ontario, B.C., Newfoundland, and Saskatchewan.
Project Looking Glass will unite Canadian enterprise, academic, and municipal groups to create innovative methods for communities to mitigate and recover from the COVID-19 pandemic. Project Looking Glass will be rolled out quickly to municipalities that have indicated their interest, and Distributive is accepting new users all the time.
If you are a municipality that is interested in implementing this platform to make more data-driven decisions, contact Distributive at [email protected] today.
CENGN is a Non-Profit Organization that provides infrastructure services to help Canadian small and medium enterprises commercialize their products and grow. Distributive is a CENGN Project Alumni company from Kingston, Ontario. CENGN is supporting Project Looking Glass by working with Distributive to provide compute support and helping test the Distributed Computer application at the scale required to mimic its projected use across the country. CENGN is proud to be able to support Distributive and by extension fight the negative impacts of COVID-19 in Canada